Application of Principal Component Analysis As A Data Reducing Technique
Keywords:
Principal Component Analysis, Principal Component, Kaiser’s Criterion, Guttman, Kaiser, Karhunen-Loeve Transform, Proper Orthogonal DecompositionAbstract
This paper is on the application of principal component as a data reducing technique on economic variables for the period of 26 years. The source of data was secondary and was collected from the Central Bank of Nigeria Statistical Bulletin. The aim is to use principal component analysis effectively and profitably to reduce the large and massive economic variables (Data) to a smaller number of PCs while retaining as much as possible of the variation in the original variables. The methodology employed Principal Component which are orthogonal in nature from the original Economic Variables. The criterion for selecting the number of Principal Component to
be extracted is the KAISER’S CRITERION which was suggested by GUTTMAN and adopted by KAISER. The result of the analysis revealed that the variables BOP, LR, and INFL have low correlation coefficient with other variables. Furthermore, results showed that the large sample size of economic variables have being reduced and the principal components are extracted in which the first Principal Component have the highest number of variables which are positively highly correlated, the second Principal Component loads positively with Crude Oil production, Lending Rate and Inflation Rate while the third Principal Component load positively with
Balance of Payment.
References
Anderson D.R, Sweeney D.J, Williams T.A Freeman J. & Shoesmith, E. (2007).“Statistics for Business and Economics”.9th edition. Thomson. ISBN-13- 978-1-84480-313-2.
Central Bank of Nigera (2008) Statistical Bulletin Golden Jubilee Edition, CBN Press Abuja.www.cbn.gov.ng/documents/Statbullentin.
asp
Dillon W.R., Goldstein M. (1984). Multivariate Analysis: Methods and Applications. 587 Pages. Wiley.
Gower J. (1966). Some Distance Properties of Latent Root and Vector Methods Used in Multivariate Analysis. Journal Storage. Biometrika Trust, vol. 53. pp:325-338.
Jeffers J.N.R. (1967); Two Case Studies in the Application of Principal Component Analysis. Applied Statistics. Journal of the
Royal Statistical Society. 16:225-236. Doi:10.2307/2985919.
Johnson R.A., & Wichern D.W. (1982). Applied Multivariate Statistical Analysis. (2nd edition). Upper Saddle River, NJ: Prentice
Hall.
Johnson R.A., & Wichern D.W. (1988). Applied Multivariate Statistical Analysis. (3rd edition) Englewood Cliffs, NJ: Prentice
Hall.
Johnson R.A., & Wichern D.W. (2002). Applied Multivariate Statistical Analysis. (4th edition), Upper Saddle River, NJ: Prentice
Hall.
Johnson D.E. (1998). Applied Multivariate Methods For Data Analysts. 567 Pages. Duxbury Press
Kaiser, H.F. (1960). The Application of Electronic Computers Factor Analysis, Education & Psychological Measurement 20, 141-151.
Doi:10.1177/00131646002000116.
Morrison D.F. (1967). Multivariate Statistical Methods, Mathematical Statistics. 338 Pages. McGraw-Hill Book Company,
New York.
Morrison D.F. (1983). Applied Linear Statistical Methods. Englewood Chiff, New Jersy,
Pentice Hall.
Morrison D.F. (2007). Multivariate Statistical Methods. McGraw-Hill Book Company,New York. XI11 +X338 S., 15 Abb., 32Tab.
O’Brien, R.M. (2007). A Caution Regarding Rules of Thumb for Variance Inflation Factors. Quality and Quantity. International
Journal of Methodology. Springer. Vol. 4 pp. 673-690. doi:10.1007/s11135-006-9018-6.
Psych J. E. (1983). Hotelling H: Analysis of a Complex Statistical Variable into Principal Components., 26:417-441.
Sam K. K. (1991). Multivariate Statistical Analysis, A Conceptual Introduction.303 Pages. Radius Press.
Stevens J. (1986). Applied Multivariate Statistics for Social Sciences. New Jersey United States of America. Lawrence Elbaum Associates, Inc.
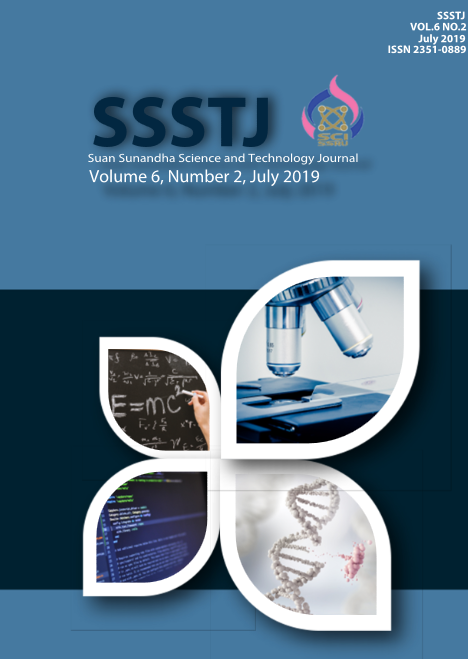