A Risk Prediction Model of Road Accidents During Long Holiday in Thailand Using Ensemble Learning with Decision Tree Approach
DOI:
https://doi.org/10.53848/ssstj.v10i2.499Keywords:
road accident, decision tree, ensemble learningAbstract
The rate of injury and death from traffic accidents during the New Year and Songkran Festival each year has high and are continuously on the increase. The researchers, therefore, has decided to study and develop a model for predicting the road accident risk during the holiday season with ensemble learning based on decision tree approach. The aim is to help reduce accidents and loss of life caused by road accidents. The dataset used in this research is traffic accidents resulting in injury and death data during the long holiday from 2008 to 2015 from hospitals across the country, accumulatively recorded by the National Institute for Emergency Medicine. This
research compared the efficiency of data classification to find the best ensemble model for predicting traffic accident risk. The methods studied included Adaptive Boosting (AdaBoost), and Random Forest, and the decision tree techniques used in the experiment were J48, ID3, and CART. The results of experiment and comparisons of classification efficiency showed that the Random Forest algorithm with J48 decision tree was the most efficient model, providing an accuracy of up to 93.3%.
References
Accident Prevention Network. (2022). Traffic accident statistics report: New Year -Songkran Festival 2022. Retrieved from http://www.accident.or.th/ index.php/2017-12-04-07-32-28/289-2565
Boonraksa, P., & Thongkam, J. (2018). Performance comparison of the road occurrence accidents prediction models using time series techniques.Journal of Technology Management Rajabhat Maha Sarakham University, 4(2), 39-46.
Chen, M.-M., & Chen, M.-C. (2020). Modeling road accident severity with comparisons of logistic regression, decision tree and random forest.Information, 11(5), 270.doi:10.3390/info11050270.
Esenturk, E., Turley, D., Wallace, A., Khastgir, S.,& Jennings, P. (2022). A data mining approach for traffic accidents, pattern extraction and test scenario generation for autonomous vehicles.International Journal of Transportation Science and Technology. doi:10.1016/j.ijtst. 2022.10.002
Markoulidakis, I., Rallis, I., Georgoulas, I.,Kopsiaftis, G., Doulamis, A., & Doulamis, N.(2021). Multiclass confusion matrix reduction method and its application on net promoter score classification problem. Technologies,9(4), 81. doi:10.3390/technologies9040081
Nedjmedine, O., & Tahar, M. (2022). Analysis of road accident factors using Decision Tree Algorithm: A case of study Algeria. 5th International Symposium on Informatics and its Applications (ISIA). M'sila, Algeria.doi:10.1109/ISIA55826.2022.9993530.
Njoku, O. C. (2019). Decision trees and their application for classification and regression problems (Master’s thesis). MSU Graduate Theses. Retrieved from https://bearworks.missouristate.edu/theses/3406
Ogheneovo, E. E., & Nlerum, P. A. (2020). Iterative Dichotomizer 3 (ID3) decision tree: A machine learning algorithm for data classification and predictive analysis. International Journal of Advanced Engineering Research and Science (IJAERS), 7(4), 514-521. doi:10.22161/ijaers.74.60
Panigrahi, R., & Borah, S. (2018). Rank allocation to J48 group of decision tree classifiers using binary and multiclass intrusion detection datasets. Procedia Computer Science, 132,323-332. doi:10.1016/j.procs.2018.05.186
Parathasarathy, G., Soumya, T. R., Das, Y. J.,Saravanakumar, J., & Merjora, A. A. (2019).Using hybrid data mining algorithm for analysing road accidents data set. 3rd International Conference on Computing and Communications Technologies (ICCCT) (pp.7-13). Chennai, India.doi:10.1109/ICCCT2.2019.8824860
Sonwongsa, R., Pinpoo, S., & Wongkhae, K. (2016).Severity analysis of car accidents during “7 dangerous days” of New Year and Songkran festival. The 12th Mahasarakham University Research Conference (pp. 39-47). Thailand.
Taamneh, M., Alkheder, S., & Taameh, S. (2017).Data-mining techniques for traffic accident modeling and prediction in the United Arab Emirates. Journal of Transportation Safety & Security, 9(2), 146-166.doi:10.1080/19439962.2016.1152338
Tanha, J., Abdi, Y., Samadi, N., Razzaghi, N., & Asadpour, M. (2020). Boosting methods for multi-class imbalanced data classification: An experimental review. Journal of Big Data,7(1), 1-47. doi:10.1186/s40537-020-00349-y
Yang, P., Yang, Y. H., Zhou, B. B., & Zomaya, A.Y. (2010). A review of ensemble methods in bioinformatics. Current Bioinformatics, 5(4), 296-308. doi:10.2174/157489310794072508
Zacharis, N. Z. (2018). Classification and Regression Trees (CART) for predictive modeling in blended learning. International Journal of Intelligent Systems and Applications, 3, 1-9.doi:10.5815/ijisa.2018.03.01
Zamzuri, Z. H., & Qi, K. Z. (2022). Classifying the severity levels of traffic accidents using decision trees. Proceedings of the International Conference on Mathematical Sciences and Statistics 2022 (ICMSS 2022).doi:10.2991/978-94-6463-014-5_17
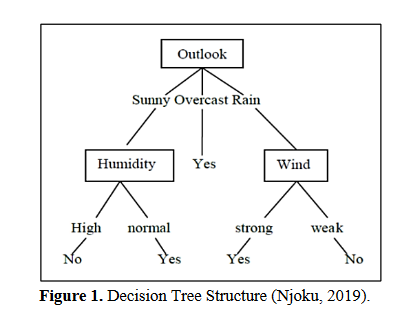
Downloads
Published
How to Cite
Issue
Section
License
Copyright (c) 2023 Suan Sunandha Rajabhat University

This work is licensed under a Creative Commons Attribution 4.0 International License.